Using SPSS for sports analytics: a case study on Fulham FC
20th June 2024
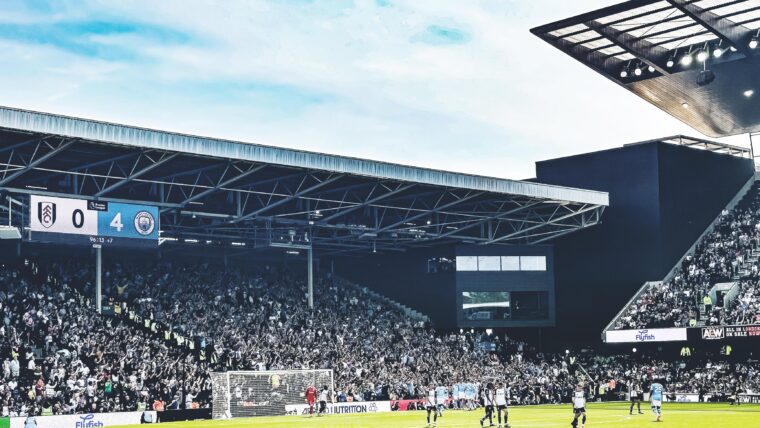
In sports, where things move quickly, data analytics has become a crucial technique to get an advantage over competitors. Although there are many analytical tools available, SPSS (Statistical Package for the Social Sciences) stands out for its ability to perform robust statistical analysis. This article will examine FC to demonstrate how SPSS may be utilised for sports analytics. As we examine this instance, we will discover how statistics might influence football plans and performance.
Introduction to SPSS and sports analytics
First, we should define SPSS and explain why it is beneficial in sports analytics. Only then can we discuss Fulham FC further. SPSS is a collection of programs that can be used to do live or batch statistical analyses. It allows you to run a variety of statistical tests and generate attractive graphs from the data you’re working with.
In the field of sports statistics, SPSS assists teams in analysing how players performed, how the team collaborated, and how games turned out. This statistical tool enables teachers and managers to make sound decisions, improve training programs, and devise successful strategies.
The importance of data in modern football
Like many other sports, football has turned to data analytics to improve performance and gain strategic advantages. With the advancement of technology, data collection has become more complex, capturing a wide range of variables, from player movements to matching numbers. But what exactly does this information include?
Types of data in football
- Player performance data: Data about how well a player did: this includes things like speed, distance travelled, passes made, shots on target, and more.
- Match statistics: Match statistics include things like the proportion of time the ball was in play, the number of corners, fouls, and goals scored.
- Biometric data: Biometric data includes things like a player’s heart rate, accident history, and how long it takes to recover from an injury.
Like many elite teams, Fulham gathers and studies this kind of information to improve their play.
Case Study: Fulham FC
Data collection and preparation
For this case study, let’s consider Fulham’s 2020/21 season. The data collection process began with gathering match statistics, player performance metrics, and biometric data. These data points were then cleaned and prepared for analysis using SPSS. Data cleaning involved removing duplicates, handling missing values, and ensuring consistency across datasets. When working on such extensive data, it can be helpful to seek SPSS help for students from EduBirdie, especially if you’re facing tight deadlines or lack experience. Professional writers from EduBirdie can assist in organising and analyzing the data efficiently, ensuring that the data is accurate and ready for further analysis. This collaboration can be crucial for students and researchers who need to meet strict deadlines while ensuring high-quality, reliable results.
Exploratory data analysis (EDA)
An experimental data analysis (EDA) was the first thing that had to be done with Fulham’s data before SPSS could be used. EDA helps you understand how the data is spread out, find trends, and spot outliers. We used SPSS to make summary statistics and graphs like histograms, box plots, and scatter plots.
For instance, a histogram of goals scored per game showed that Fulham got fewer goals on average than other Premier League teams. This new information led to more research into the team’s offensive plans and how well players were doing when they had the ball in scoring position.
Hypothesis testing and statistical analysis
Next, we used SPSS to test our hypotheses and figure out what factors affect the results of Fulham’s games. One idea was that player health has a big effect on how well they do in matches. We used biometric data as the independent variable and match outcomes as the dependent variable in a regression study to see if this was true.
The regression data showed that there was a strong link between how fit a player was and how well they did in a match. In particular, players with higher fitness measures made more useful contributions during games, which helped their teams win.
Advanced analytics: predictive modeling
Building predictive models
We were able to make predictive models for Fulham Football Club using SPSS’s powerful analytical tools. We used methods such as logistic regression and decision trees to guess how the matches would end based on things like player performance measures and the strength of the other team.
For example, a logistic regression model was made to figure out how likely it was that Fulham would win a game. The model used factors such as average player speed, possession percentage, and the results of recent games. Cross-validation methods were used to prove that this model was accurate at making predictions, which made it reliable.
Implementation and results
The predictive models gave Fulham’s coaching team useful information they could use. The team could tailor their training sessions, choose the best player lineups, and come up with effective match plans if they knew the main factors that affect how games turn out. Because of this, Fulham’s performance measures got better, such as their win rate going up and their players being more efficient.
Visualisation and reporting
One of the strengths of SPSS is its ability to create compelling visualisations. For Fulham, we generated dashboards and reports that highlighted key performance indicators (KPIs). These visualisations included:
- Heat maps: Showing player movements and positions during matches.
- Performance dashboards: Summarizing key metrics like goals, assists, and defensive actions.
- Trend analysis graphs: Illustrating performance trends over the season.
These visual tools enabled the coaching staff to quickly grasp complex data insights and make data-driven decisions.
Conclusion
The case study of Fulham FC demonstrates the profound impact of using SPSS for sports analytics. By leveraging SPSS’s powerful statistical analysis and visualisation capabilities, Fulham was able to gain valuable insights into their performance, leading to strategic improvements and better results on the field.
In the modern era of football, data analytics is not just a luxury but a necessity. Teams that effectively harness the power of data, like Fulham FC, are better positioned to succeed. Whether you’re a data analyst, a sports enthusiast, or a football manager, understanding and utilising tools like SPSS can significantly elevate your approach to the beautiful game.